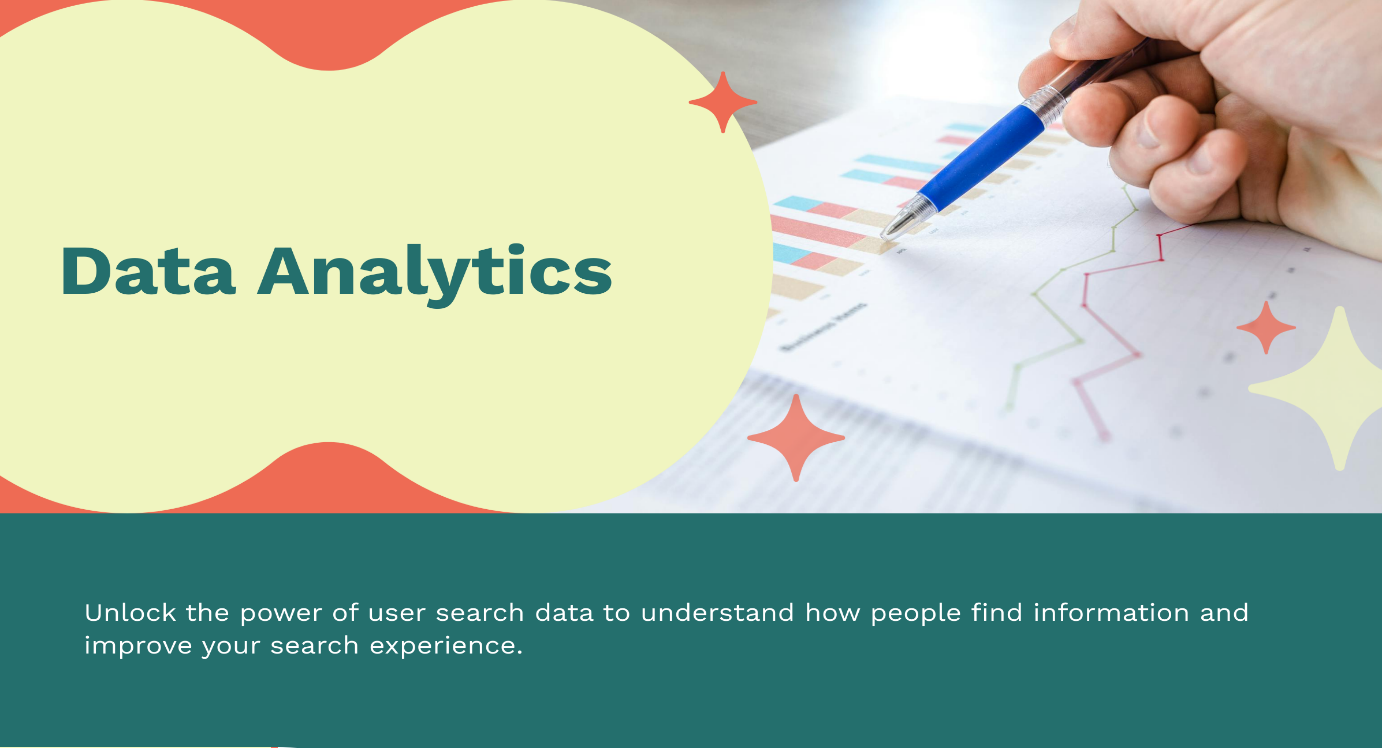
Search analytics is a term that people nowadays mostly associate with digital marketing and SEO. It is useful because it uncovers the trends and patterns in user behavior, and that itself holds valuable data. It tells the story of what people are searching for and what queries they use to search for it.
Whether you're running a business website or managing an internal company platform, these insights can make a real difference. For businesses, it helps them show up better in search results and understand what their customers want. Inside companies, it makes their internal search systems work better, helping employees find what they need faster.
In this article, we'll break down what search analytics is all about, look at different ways it can be used, and check out the main tools that help collect and make sense of all this search data. We'll also see how analyzing search patterns helps make smarter decisions, both in marketing and within organizations.
What is Search Analytics?
Simply put, search analytics is the practice of analyzing data generated from user searches on websites or search engines. When people use search, they leave behind a trail of valuable information - what words they type in, which results catch their eye, and how they move through the search results.
The data shows key information like:
- The exact words and phrases people type into search boxes
- Which search results they click on (and which they ignore)
- How long they spend looking at results
- Whether they find what they're looking for or try different searches
This information reveals what's really going on in people's minds when they search. It tells you:
- What problems they're trying to solve
- The language they naturally use to describe what they want
- Whether your content matches what they're looking for
- If your search system is actually helping them find answers

For businesses and organizations, these insights are invaluable. They help improve everything from website content and product descriptions to the way internal search systems work. Instead of guessing what people want, you can see it directly in the data.
What are The Key Components of Search Analytics
Key Components of Search Analytics
At its core, search analytics relies on several essential components and metrics that work together to provide a complete picture of search behavior. Here are the main elements:
Search Queries and Keywords
- The actual terms people enter into search boxes
- How these terms vary and change over time
- Which keywords lead to successful searches
- Common misspellings and variations
User Behavior Metrics
- Click-through rates (CTR): how often people click on specific results
- Time spent on results pages
- Bounce rates: when users leave immediately
- Search refinements: when users modify their original search
Technical Performance Data
- Search response times
- Search result relevancy scores
- Failed searches and zero-result queries
- System performance under different loads
Advanced Analytics Elements
- Search patterns across different devices
- Geographic location data
- Time-based trends and seasonality
- User journey mapping through search
These components combine both user behavior data and technical performance metrics. Tools like Google Analytics, along with specialized search analytics platforms, collect and process this information. The resulting data helps organizations understand not just what people search for but how effectively their search systems serve these needs.

Similarly to large search engines like Google, search data can be collected and analyzed in any kind of search engine. Data collection methods include search log analysis, user session tracking, and query pattern monitoring. Enterprise search platforms come with built-in analytics tools that help organizations track internal searches and user behavior.
These tools measure internal search volumes, document access patterns, and failed searches. This helps organizations understand how employees use their search systems, which resources are most accessed, and where information might be hard to find. Unlike public search data, this analysis focuses on improving internal productivity and information access.
What Insights Can You Get with Search Analytics
In essence, search analytics enables search engines to fine-tune their results. The same data that marketing experts so carefully analyze to show you the right ads (that you will still probably hate) can be used for something that will actually improve your search experience and provide valuable insights into what kind of search results people find useful and satisfy their search intent.
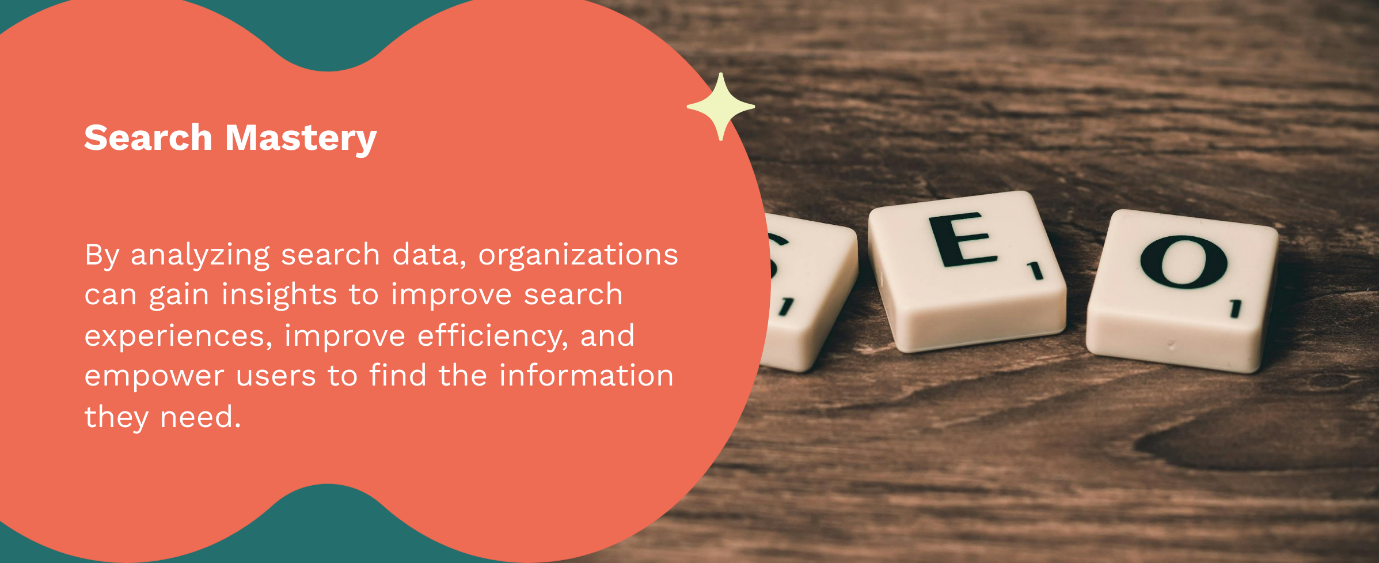
The same principles that make search analytics valuable for public search engines apply to enterprise search systems. When employees search for information within company platforms, they generate data that reveals what knowledge they need and where they struggle to find it.
This internal search data tells an important story about how your organization works. When multiple employees repeatedly search for the same information, it might indicate a gap in your documentation. When searches fail to return useful results, it shows where your knowledge base needs improvement.
Organizations can use these insights to make practical improvements. For example, if employees frequently search for specific procedures, that's a clear signal to create better documentation or training materials for those topics. If certain resources are consistently hard to find, it might be time to reorganize how that information is structured or employ a method like vector search that can sort through the unstructured data.
Search Analytics with Akooda
Search analytics continues to shape how we think about search functionality, both in public search engines and enterprise systems. While collecting and analyzing search data might seem primarily focused on marketing and SEO, its real value lies in continuously improving the search experience for users.
When search systems analyze user behavior, they learn what makes a search result truly valuable. This understanding enables them to improve result relevancy over time, moving beyond simple keyword matching to understanding actual user intent. The same principles apply whether you're optimizing a public website or an internal enterprise search system.
Akooda Enterprise Search takes this concept further by combining search analytics with advanced machine learning capabilities. The platform learns from every search interaction, understanding not just what users search for but what kind of results actually solve their problems.
For example, when a user repeatedly searches for specific types of information using detailed queries, the system learns these preferences. After recognizing the pattern, it can provide relevant results even with shorter, related searches.

In addition, Akooda can also search through unstructured data and provide relevant results that satisfy user intent.
This combination of search analytics and machine learning represents the future of search technology. Instead of treating each search as an isolated event, modern search systems build understanding over time. They remember user preferences, learn from behavioral patterns, and continuously adapt to provide more relevant results. This evolution in search technology means users spend less time searching and more time working with the information they need.