While generative AI has revolutionized the modern workplace, 63% of organizations struggle with proper implementation. Business leaders face the challenge of transforming AI's potential into practical workplace solutions. Success with generative AI requires more than technical knowledge—it demands a strategy aligned with business objectives.
Organizations require a structured framework to guide their generative AI implementation. This guide outlines the key steps for building a foundation and progressing from initial assessment to full deployment, including methods to track ROI and address common challenges.
Building the Foundation for AI Integration
Successful generative AI implementation begins with a strong foundation. Global spending on AI implementation strategies is projected to reach $337 billion by 2025 and $632 billion by 2028, underlining the importance of getting the fundamentals right.

Assessing Organizational AI Readiness
Initial implementation requires a comprehensive readiness assessment. With only 54% of AI projects moving from pilot to completion, organizations must evaluate four key factors to avoid failure:
- Data Management Capabilities
- Technical Infrastructure Readiness
- Team Expertise and Skills
- Governance Framework Maturity
Defining Strategic Implementation Goals
AI initiatives require clear business objectives. Two-thirds (67%) of projected AI spending in 2025 will focus on embedding AI capabilities into core business operations. Implementation goals should address both short-term operational efficiencies and long-term strategic advantages.
Creating a Technology Infrastructure Blueprint
Infrastructure planning must accommodate current needs and future growth. Industry analysis indicates that generative AI workloads are expected to increase from 7.8% to 36% of the overall AI server market by 2027. Essential steps for infrastructure development include:
- Reviewing existing data storage and computing capabilities
- Evaluating cloud vs. on-premises solutions
- Planning for scalability and performance requirements
- Implementing strong security measures
- Establishing data governance protocols
The foundation requires adaptability, as 53% of enterprises plan to start with pretrained models before enhancing them with enterprise data. This approach necessitates flexible infrastructure supporting both initial implementation and future growth while maintaining robust governance practices.
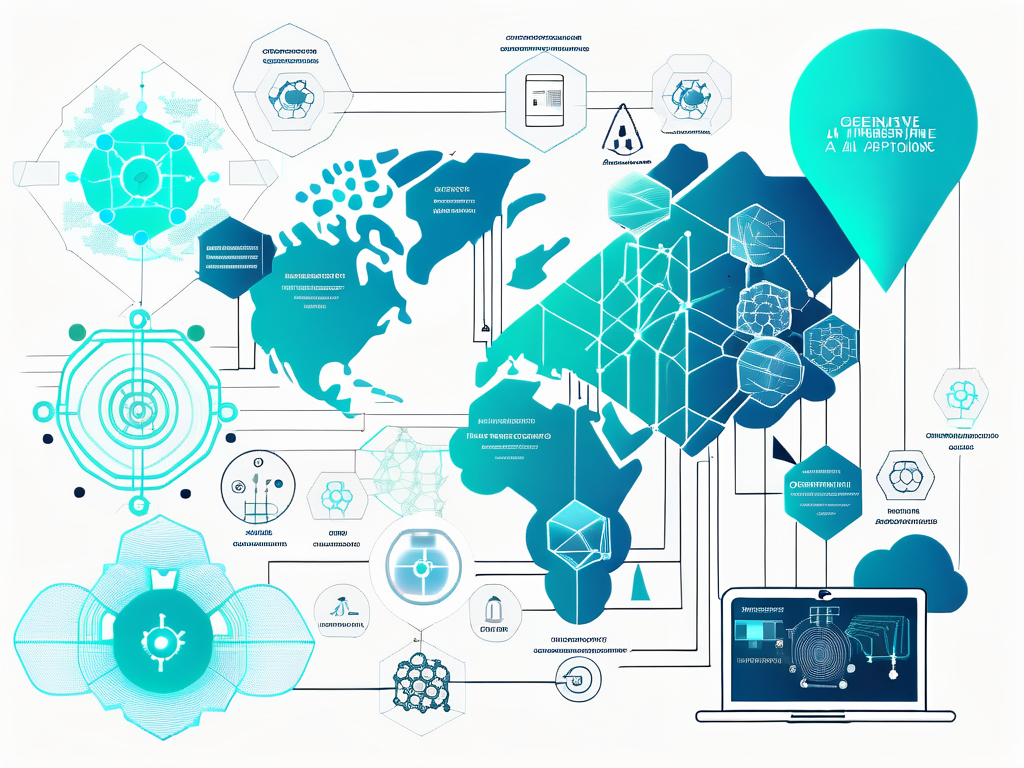
Developing a Phased Implementation Strategy
Phased implementation is crucial for managing risks and ensuring successful generative AI adoption. Organizations using phased implementation are 10 times more likely to achieve significant financial benefits from their AI investments.
Pilot Program Design and Execution
Implementation begins with selecting specific use cases aligned with business objectives. Successful pilot programs typically run for 8 weeks, utilizing structured sprint cycles for development and testing.
Scaling Successful AI Initiatives
After proving pilot project success, organizations can expand implementation across the enterprise. This stage requires:
- Performance tracking and feedback loops
- Integration with existing systems and processes
- Regular model updates and optimization
- Staff training and support programs
Organizations utilizing AI with performance data consistently achieve better results compared to traditional approaches.
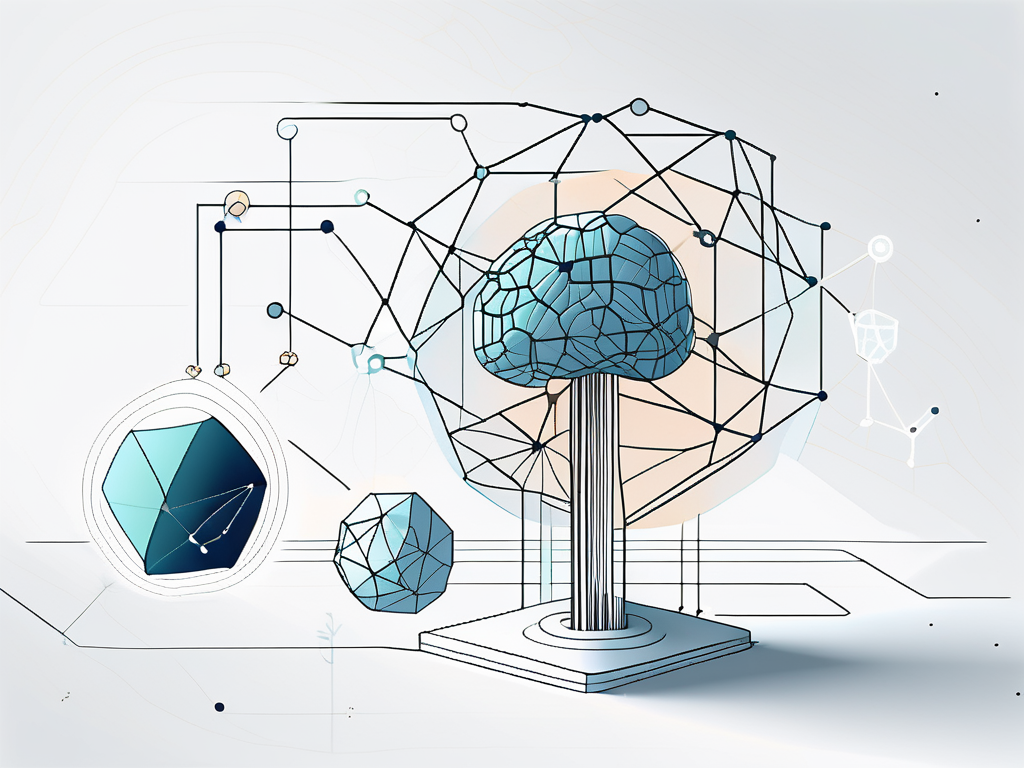
Measuring and Optimizing Performance
Performance tracking should encompass both technical success and business outcomes. Successful AI projects demonstrate improvements in several areas:
- Efficiency Gains: Reduced process time and improved resource utilization
- Accuracy Improvements: Enhanced precision in task completion
- Cost Savings: Reduced direct and indirect costs
- User Adoption Rate: Increased employee utilization and satisfaction
Clear metrics help identify improvement areas and ensure AI projects deliver tangible value. Organizations achieving substantial financial benefits from AI investments often revise their success metrics.
Establishing AI Governance Framework
A robust governance framework is essential for generative AI implementation, ensuring long-term success and risk mitigation. Currently, 46% of business and cybersecurity leaders express concern about advanced adversarial capabilities in generative AI, while 55% of inputs to generative AI tools contain sensitive or personally identifiable information.
Data Privacy and Security Protocols
With an 80% increase in file uploads to generative AI tools, robust security protocols are essential. Recommended security measures include:
- Data sanitization and encryption protocols
- Access control mechanisms
- Regular security audits
- Secure deployment pipelines
- Real-time monitoring systems
Ethical Guidelines and Compliance Measures
Compliance forms the cornerstone of responsible AI implementation. The NIST AI Risk Management Framework, released in January 2023, provides a consensus-driven approach for building trustworthy AI systems. Implementation should align with these guidelines while ensuring transparent and accountable AI operations.
Risk Management Strategies
Risk management requires a comprehensive and proactive approach. Studies indicate that less than half of organizations adequately address risks associated with generative AI technologies. Three key areas require attention:
- Operational Risk Management: Continuous monitoring and response systems
- Data Protection: Safeguarding proprietary information and preventing unauthorized access
- Compliance Risk: Regulatory alignment
Organizations with effective AI governance strategies build greater trust with stakeholders, including customers, investors, and regulators.
Managing Organizational Change
Successful generative AI implementation requires understanding the human element. With 60% of industry respondents expecting significant disruption from generative AI, the human aspect of transformation becomes critical.
Employee Communication and Training Programs
Transparency is key to successful AI adoption. Currently, 54% of employees lack awareness of their companies' AI utilization. Comprehensive training programs should focus on:
- Technical skill development
- Practical AI application scenarios
- Ethical considerations
- Best practices for AI tool usage
- Continuous learning opportunities
Addressing AI Anxiety and Resistance
With 39% of workers concerned about AI-related job displacement and 88% distrusting their employers regarding AI technology understanding, organizations must address the trust gap. A multi-faceted approach to reducing resistance, including readiness sessions and hands-on training, has proven effective. Organizations achieve 75% higher employee enthusiasm about AI through maintaining transparency and positioning AI as a tool for professional growth rather than worker replacement.
Building Cross-functional AI Teams
Diverse expertise drives successful generative AI implementation. Cross-functional teams typically include:
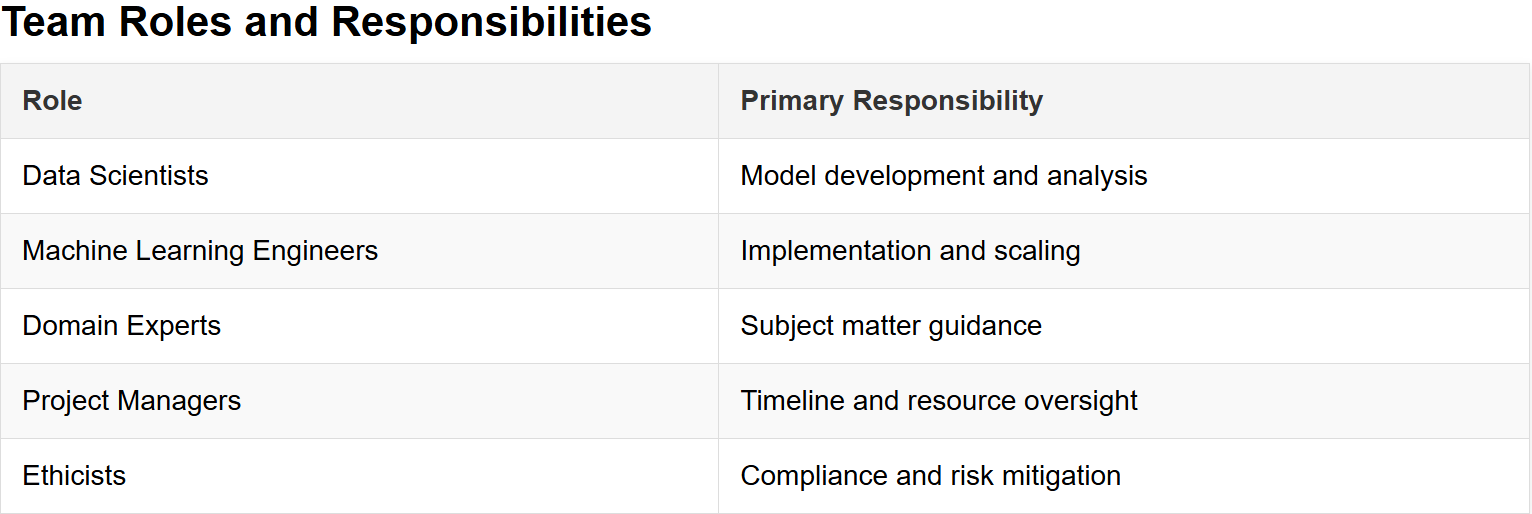
Organizations with effective cross-functional teams are 28% more likely to succeed in change initiatives. These teams maintain open communication channels and regular collaboration, creating an environment conducive to innovative solutions.
Conclusion
Successful generative AI implementation requires careful planning, robust governance, and effective change management. The path to success encompasses five key elements: comprehensive readiness assessment, strategically aligned objectives, phased deployment, governance frameworks, and stakeholder engagement.
Organizations taking this structured approach can maximize benefits. Research demonstrates that enterprises with proper governance and change strategies achieve superior efficiency, accuracy, and cost savings while maintaining employee trust.
The key lies in balancing technological advancement with human needs. Effective communication, targeted training, and cross-functional collaboration create an environment where AI enhances human potential rather than replacing it. Data shows that organizations focusing on these elements achieve higher adoption rates and stronger returns on AI investments.
This comprehensive strategy enables organizations to realize AI's full potential while managing associated risks. Through proper planning and execution, business leaders can transform AI theory into practical workplace solutions that deliver meaningful results.