Artificial intelligence (AI) includes predictive and generative forms, each playing unique and often complementary technological roles.
Predictive AI specializes in analyzing and interpreting existing data. It looks for patterns and historical information to make forecasts and predictions. This AI type is focused on understanding past and present data to inform future decisions. It’s about using what we know to predict what might happen next, offering a data-driven basis for planning and decision-making.
Generative AI, in contrast, is about creating new content. Rather than analyzing data, it uses learned patterns to produce original outputs, like text, images, or music. This AI type moves beyond the limits of existing data, applying what it has learned to invent and design things that didn’t exist before. It represents the creative side of AI, pushing the limits of what can be achieved through technology.
When these two types of AI work together, they open up new possibilities. Predictive AI’s insights into data patterns provide a strong starting point for generative AI. With this base, generative AI can create innovative and relevant solutions and content. This collaboration enhances both: predictive AI becomes more dynamic, and generative AI is informed by solid, data-driven understanding. Together, they cover the full range of AI’s abilities, from making sense of the world to creating new and exciting possibilities.
How Generative AI Works
Generative AI learns from a vast amount of existing data (like texts, images, or music) and then uses this learning to create new, original content that mimics the input data. It does this by recognizing patterns and structures in the data it has learned from and then generating new content that follows these patterns. For instance, if it's trained on thousands of paintings, it can create new paintings that look like they could have been made by those artists.
Generative AI often uses complex algorithms like Generative Adversarial Networks (GANs), where two parts of the model work against each other – one generates content, and the other evaluates it. This process continues until the generated content is of high quality.
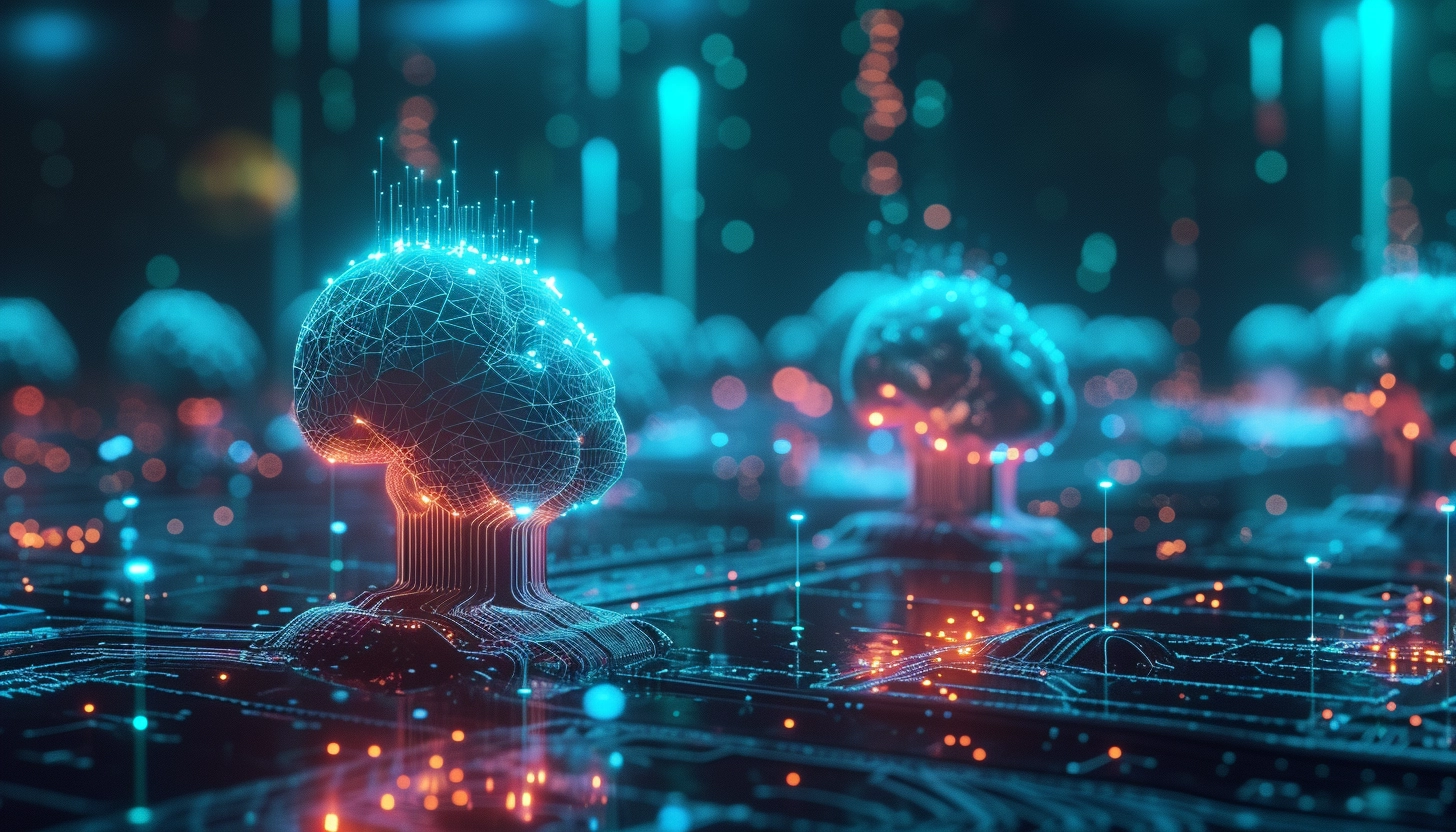
How Predictive AI Works
Predictive AI, a well-established technology, aids organizations by analyzing historical data to make data-driven predictions. Its primary purpose is to identify patterns in past data, enabling businesses to forecast future trends, behaviors, and outcomes.
This capability of predictive AI aided in developing tools like enterprise search and insight engines that help with risk management and optimizing operations, as they provide insights that help organizations anticipate and prepare for future scenarios. By leveraging predictive AI, companies can make more informed decisions, resulting in much higher efficiency and competitiveness in their respective markets.
Generative AI Takes the Spotlight
Generative AI has been around for a while, but its capabilities were far more limited initially. The recent breakthroughs, especially in models like ChatGPT, have hugely improved its capabilities. These advancements are due to improvements in algorithms, increased computational power, and access to larger and more diverse data sets.
Today, generative AI is taking the spotlight because of its ability to create highly realistic and sophisticated outputs. From writing essays to creating art, it's showing potential across various fields. This has sparked much interest and excitement, leading many to believe that generative AI will play a key role in future technological advancements. It's not just about its creative abilities but also about how it can solve complex problems and support innovation in numerous industries.
Synergy of Predictive and Generative AI
To understand the fusion of generative and predictive AI, it's crucial to first explore their similarities and then their distinct focuses, which ultimately lead to their synergistic potential.
Both generative and predictive AI are rooted in the field of machine learning (ML) and rely on similar underlying technologies:
- Both utilize a range of machine-learning algorithms to process and learn from data. This includes neural networks, a type of AI that mimics the human brain's structure and function.
- Both are heavily reliant on data. They learn from data patterns – generative AI to create new content and predictive AI to forecast future events.
- Deep learning, a subset of ML, plays a significant role in both. This involves layered neural networks that analyze different aspects of the data, providing a depth of understanding and functionality.
Despite these similarities, generative and predictive AI have different focuses:
- Generative AI is about creating new, original content or data, whereas predictive AI is about forecasting future events based on historical and current data.
- Generative AI leans more towards creative tasks – generating text, images, music, etc. Predictive AI, in contrast, is more analytical, focusing on pattern recognition, trend analysis, and decision-making based on data analysis.
When these technologies work together, they unlock new possibilities:
- It leads to improved data analysis and content relevance. Predictive AI can analyze customer preferences or market trends, providing insights that generative AI can use to create highly relevant and targeted content. For example, in marketing, predictive AI can identify what kind of content resonates with a particular audience segment, and generative AI can then produce content that resonates with the specific target group.
- It enables advanced simulation and forecasting. Generative AI can simulate various scenarios based on data analyzed by predictive AI. This is particularly useful in fields like urban planning or environmental modeling, where predictive AI forecasts future scenarios (like traffic patterns or climate changes), and generative AI simulates the impact of different response strategies.
- It makes personalization and customization so much easier and relevant. In customer service, predictive AI can anticipate customer needs or problems based on past interactions, while generative AI can create personalized responses or solutions, enhancing customer experience.
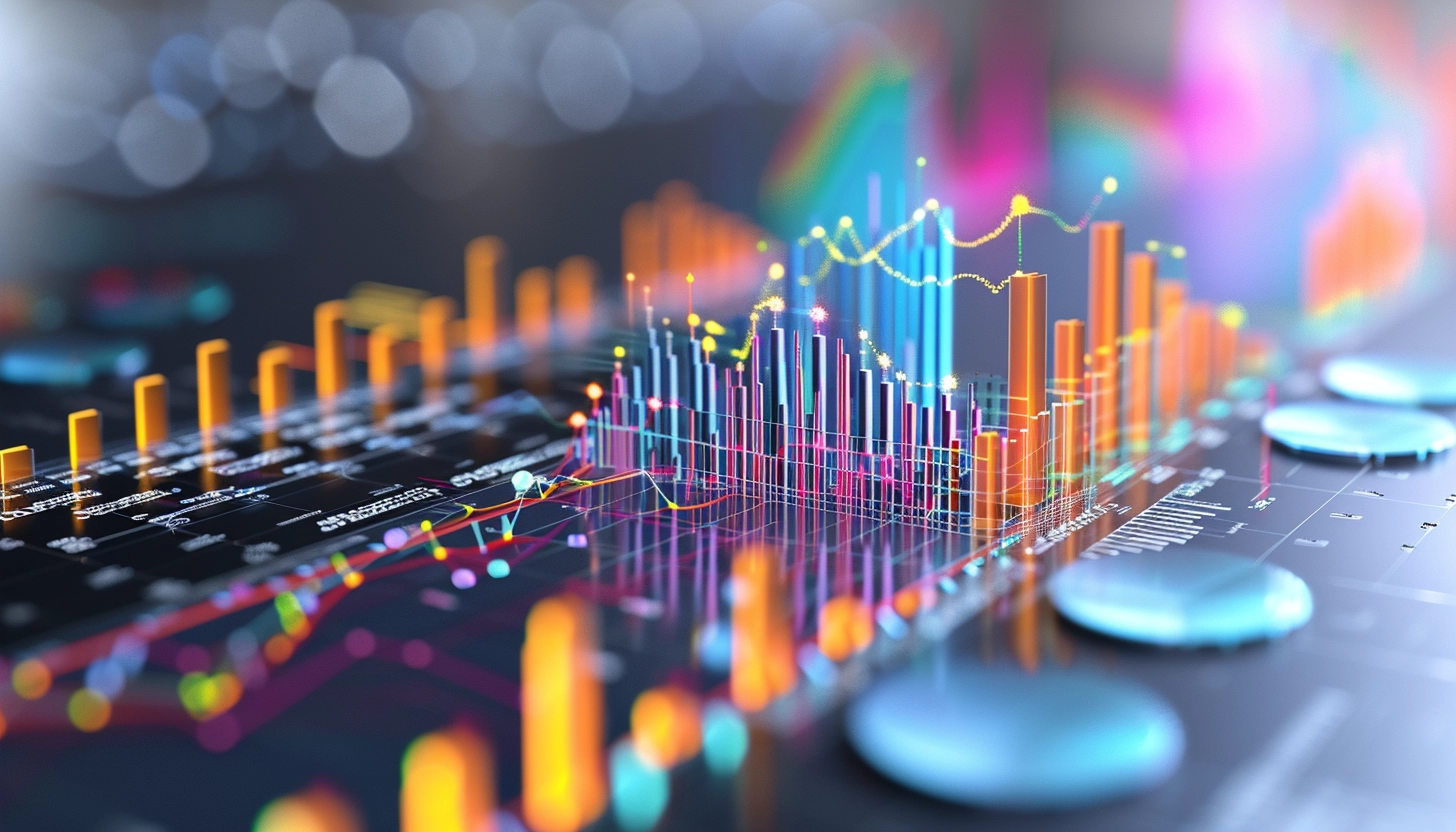
Future Prospects of AI Technology
As Predictive AI and generative AI continue to develop, their integration slowly leads to progress in areas crucial for planning, knowledge-sharing, and resource management within organizations. Enterprise search engines are reaching a whole new level of interactive Q&A experience that they are being labeled as enterprise search platforms.
This ultimately means that in times to come, tools that assist in the decision-making process will surely take the spotlight. By combining predictive insights with generative creativity, businesses can approach advanced decision-making considering a broader range of variables and potential outcomes.
We can also expect improvement with innovative problem-solving platforms. With the ability to forecast challenges and generate solutions, this fusion could lead to innovative approaches to tackling complex problems in healthcare, environmental management, and more.
In summary, the fusion of generative and predictive AI – grounded in their shared machine learning foundations but distinct in their creative versus analytical focuses – offers a powerful synergy that will open a whole new world of data utilization and management options.