Financial analysis and investment management are both incredibly serious and competitive fields and as such, the methods involved in each field are becoming increasingly sophisticated.
What that means essentially is that each step in the decision-making process needs to be fed with the appropriate amount of high-quality data. This is simpler said than done, as the sheer abundance of data makes it hard to select what is relevant, what should, and what should not be included in the financial decision-making models.
There are various data sources that can feed the process of financial analysis and investment research. Market trends, customer behavior, economic indicators, marketing campaign engagement levels, global trends, and many more.
Gaining valuable insights and spotting patterns is not a straightforward process that can be mathematically repeated across different scenarios, as it often requires creativity and knowing where to look and how to look at the available data.
One thing is certain: staying competitive in these fields includes collecting an increasing amount of data.
Collecting Data Is Only the First Step in the Research Process
However, simply collecting data from a lot of diverse data sources is only the first step in the research process.
This brings forward two main challenges in the way of making data-informed financial analysis and investment management decisions:
- Making all of the data easily searchable and available
- Getting actual value and extracting insights from these huge data sets
Business today have opportunity to feed their research process from a variety of sources. They can focus on customer behaviour, market trends, social media, business model analysis… Each insight is piece of puzzle that helps paint the whole picture in making informed decisions. The more pieces of a puzzle available, the better chance of actually making a good financial decision.
Achieving this is not a straightforward process. Alongside collecting a lot of diverse and relevant data sources (one also needs to know where to look), researchers also need to actually derive actionable insights from the said data. That takes a lot of effort and experimentation,
Insight might be hidden in the various cross-sections of different data sources, some of which might not even be purely statistical but also related to more abstract concepts like “social media sentiment.”
What this essentially means is that the process of making informed financial decisions requires careful research and looking for insights at various cross sections. For example, you could search for financial insights by combining the cross-section of these data sources:
- Economic indicators + Social media sentiment + Company financial reports
- Satellite imagery + Weather data + Crop yield forecasts
- Patent filings + R&D spending + Industry growth forecasts
These combinations are infinite, and which combination (or combination of combinations) will provide the best answer depends on the question you ask.
While you are looking for insights, it's necessary to address the two challenges we mentioned above.
First, Researchers need to have all of the necessary data at hand, easily searchable and navigable to ensure that no relevant piece of information stays forgotten.
Second, they need to have the necessary means to extract the value from data and quickly analyze, summarize, and extract meaning from data sets.
This essentially calls for having all the relevant data easily available and having the right tools to make it valuable and extract meaningful insights, which can be achieved by implementing an enterprise search for financial analysis.
Making Data Easily Available and Making the Best Use of It

In 2024, when we talk about streamlining tasks related to knowledge management and data work, we will necessarily focus on implementing generative AI solutions.
Large language models (LLMs) have so far demonstrated significant capabilities when it comes to data processing, as they can quickly summarize large datasets, identify patterns, and generate insights from cross-sectional analyses of diverse data sources.
They can even analyze user sentiment on social media and derive essential conclusions from surveys, customer feedback, and reviews.
With all those capabilities, LLMs can be used to automate various stages of research and insight generation that used to be very labor-intensive and allow the researchers to base their decisions on as much relevant data as is available.
This brings us to the second important point- making data available.
The AI tools can be very efficient, but the quality of generative AI output will heavily depend on the quality of data it can work with. Human input is still extremely important because it's still the job of data workers to decide which data will be used in research.
So, in order to create an environment where employees can find all the necessary and relevant data across different business departments, databases, communication channels, and different tools, businesses need to utilize search engines that can search across all these different data sources and collect all the necessary information.
Given the abundance of data, many businesses have also decided to set up automated report generation, risk assessment, and sentiment analysis across different data sources. The goal is to give employees as much readily available and digestible information that they can easily utilize in their daily workflow and feed their thought process.
The question that then arises is: How to bring together this readily available business data with generative AI capabalities.
Using Enterprise Search for Financial Analysis and Investment Management
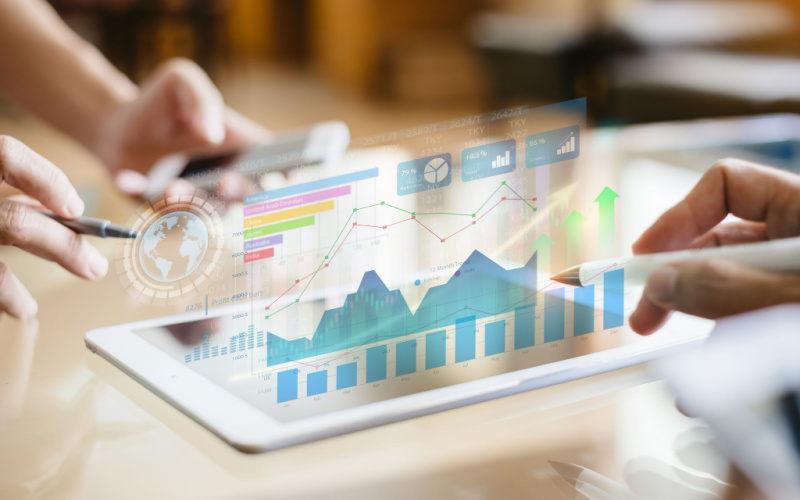
One solution that can help tackle both the integration of business data and bringing it together with generative AI capabilities is enterprise search software.
This software can be quickly implemented to create a unified and easily searchable database containing all of the diverse data sources often scattered across different data silos and connect it with large language models through APIs. When this integration happens, AI is then able to provide quick summaries, help generate visualizations like graphs and pie charts, identify patterns, and uncover insights across various data sections.
One of the biggest advantages of enterprise search is their ability to understand semantic meaning and go beyond simple keyword matching.
To illustrate this through example, we can imagine an employee querying: "Give me all the sales data for product X in Q3 2024." With this query, the search engine will scan all the relevant databases, communication channels, tools, and any other available data sources.
It can even learn based on previous interactions, so when an employee uses such tools once to collect all the relevant data for a report on sales performance on certain products, enterprise search can remember the data sources the employee used to collect information and access it next time they query for similar information.
Integration of enterprise search with generative AI allows financial analysts and investment managers to quickly access and analyze vast amounts of data from all the relevant sources. This synergy can drastically speed up the research process and offer one of the most tangible ways to measure return on investment (ROI) quickly after enterprise search implementation.
Professionals can then focus on high-level analysis and setting up new and creative ways to automate the data research process and gain new insights, and with a combination of enterprise search and generative AI, they can put their theories on the test faster than ever and check if there are any significant patterns and connections that could lead to improved financial decision-making.
How Can Akooda Help?
Akooda's AI-powered enterprise search platform offers a perfect solution for creating an ideal framework for financial analysis and investment management.
Akooda provides insights across financial data, client interactions, and market trends by integrating with existing SaaS tools. With over 50 pre-made connectors, Akooda offers one-click integration for the most widely used business tools and apps, with the option to integrate with industry-specific and custom-made tools as well.
Because it integrates with generative AI powered by Google LLM (Gemini), it enables quick summarization of complex financial documents, the creation of reports, and automation of the most labor-intensive parts of the data research process.
The end result is creating an environment where all the employees can ask any question and get all the answers, as they have all the relevant data at their fingertips, ready to be utilized to the maximum extent to make informed decisions on all levels of financial decision-making.