Knowledge management (KM) is essentially related to the processes of capturing, organizing, and sharing organizational knowledge. As such, the core of KM is based on data categorization, data analysis, processing, synthesizing knowledge, and information retrieval. The good news is that all of those processes can be made a lot easier and more efficient with AI assistance and automation.
We are well past the point where this is considered big news or controversial, as AI tools have already proven themselves to be extremely effective in practical terms and tasks related to finding information, organizing data, extracting insights from large datasets, and automating routine KM tasks.
Benefits of Using AI for Knowledge Management
Knowledge management is a branch of work that unambiguously shows the clear benefits of using AI. First of all, AI software solutions are providing the enterprise with much more effective and accurate search capabilities (vector searching) that are shown to reduce the time employees spend looking for information by around 35%.
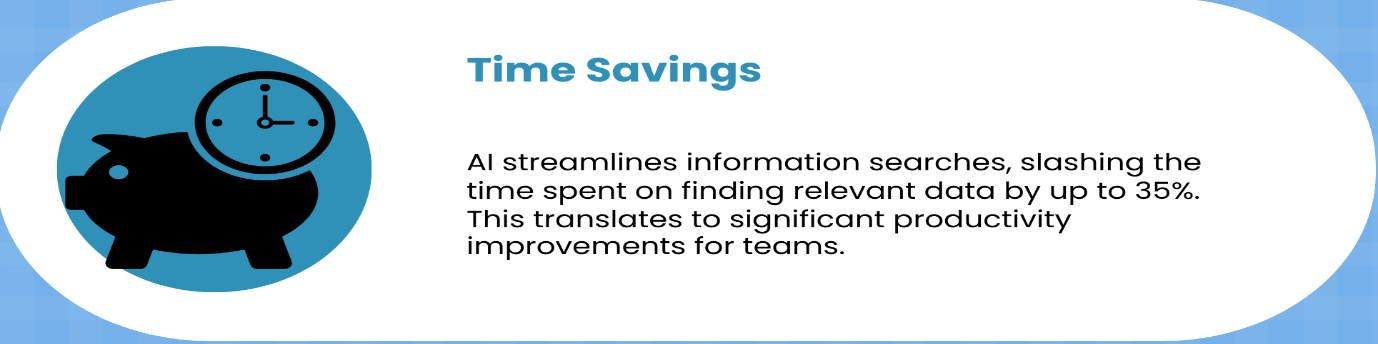
The speed of information retrieval only scratches the surface of what becomes possible with AI in knowledge management. The end game here is to create an environment where all of the data is quickly accessible, relevant, and easily utilized.
This undoubtedly relates to increased productivity and better decision-making across the organization. However, It might be harder to put the return on investment in actual numbers, as "better decision-making " and "increased productivity "might be a bit abstract terms to measure ROI.
Luckily, using AI in knowledge management also offers tangible metrics to measure ROI. It was estimated by Ventana research that in 2023, data workers spent around 69% of their time on data preparation tasks.
People who have done it themselves know how daunting, repetitive, and labor-intensive data preparation tasks can be. These tasks mostly revolve around cleaning, transformation, and organization of data, all of which can be largely automated with the help of AI software. Human input is needed only to set up initial parameters and criteria.
This is where businesses can measure the direct impact on ROI, as the time employees spend on manually organizing data can be reduced between 30% and 70%.

AI in Knowledge Management Also Enables New Features
Now that we have established that AI drastically speeds up traditional workflows for organizing KM systems, it must be said that it also brings many new features to the table.
Knowledge bases are fertile ground for implementing a variety of solutions to utilize the data. The market of AI-augmented software solutions is brimming with innovations and there is a constant influx of new and creative ways to utilize AI in order to extract the maximal value from organizations data.
Some of the most useful and widely adopted features include:
Automated summarization:
This is an incredibly handy feature that can summarize the most essential takeaways from large datasets. This feature is now often implemented in various enterprise search solutions.
Dynamic report generation:
Along with summarization, another feature often desired when you work with the data is the ability to create customized reports with various graphs and visualization, which can quickly turn a bunch of data scattered across different data sources into a manageable graph or a pie chart.
Pattern recognition:
This one also takes a lot of weight from the shoulders of data workers. With some good prompting, it’s easier than ever to set up AI to analyze data and identify patterns and trends. Employees simply need to provide the sample and ask if there is any repetition of patterns they suspect exist in the data. In addition, AI can even spot patterns that researchers might miss.
Personalized search results:
Machine learning and personalization enable AI to learn employee behavior over time. Over the course of repeated interactions, it will recognize each employee's most searched queries, the data they are looking for, and even how they prefer to summarize or visualize it. This results in employees getting the contextually relevant data needed in their usual workflow.
Intelligent data retrieval:
With vector searching and Retrieval Augmented Analytics, data retrieval goes far beyond simple lexical matching. It's now possible to input complex queries that will be understood by their context, which, in turn, results in search engines retrieving data from multiple different sources if any of them contains relevant data required to provide a complete answer.
Predictive analytics:
This one is related to pattern recognition, but it is nonetheless a creative approach to utilizing AI for data analysis, as it can forecast future trends based on historical data.
Natural language processing for unstructured data:
Summarization goes beyond a simple numbers game. With natural processing capabilities, it is now possible to extract key takeaways even from text-based sources of unstructured data. This is mainly achievable with the usage of large language models (LLM) like ChatGPT or Gemini, and if your data retrieval system integrates with LLMs, it is possible to quickly extract insights from large volumes of text. No more endless sentiment analysis of email or social media.
Automated data cleansing:
This is a tiresome, repetitive manual task for data workers that can easily be automated. AI can easily identify and correct errors or inconsistencies in datasets. It can also detect all sorts of anomalies and unusual patterns that may indicate issues with the quality of data at hand.
Organizational chart and knowledge graph creation:
Another feature implemented in knowledge management software is the creation of knowledge graphs and organizational charts. These features utilize AI to present complex networks of interrelated information or communication chains.
Automated tagging and categorization:
This also used to be tiresome and task-intensive, involving repetitive manual labor. Luckily, today, it's almost completely automated, as AI can easily classify and organize data with minimal human input.
Chatbots for knowledge retrieval:
Chatbots are being widely implemented to answer a wide range of customer queries that can be easily answered by accessing company databases, most notably inventory data. AI chatbots easily answer many of the user's questions, and we often see them talking about product features, availability, warranty, and many other features.
Cross-lingual information retrieval:
Another handy feature is that it’s not uncommon for many organizations to have data in multiple languages, especially multinationals. This presents no barrier for AI to find important relations and insights and provide relevant data in multiple languages.
Knowledge Management with Akooda
The first thing that Akooda does is create a unified search repository that contains all of the data relevant to the organization's workflow. In doing so, Akooda relies on the latest technology in RAG (Retrieval Augmented Analytics) to provide a contextually relevant answer and understand the relations between different data points in order to ensure that no data remains hidden if it's relevant to a user query.
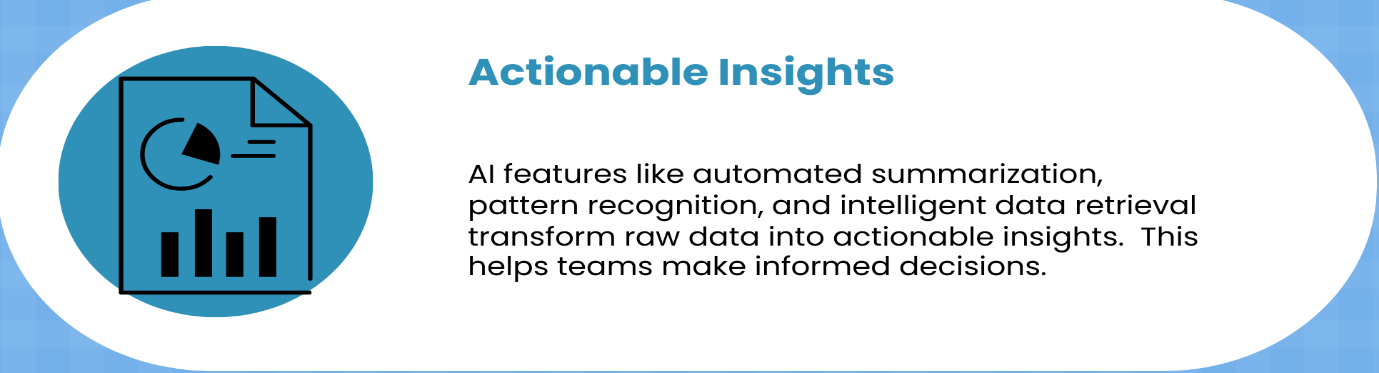
However, easily searching and navigating large databases is only one part of today's knowledge management practices. Enterprise search engines provide a great opportunity to combine relevant business data with generative AI capabilities.
Akooda integrates with Google Gemini and offers a direct link (through the API) to using the benefits of generative AI with data relevant to employees' everyday workflow. It allows for the creation of summaries, suggestions, visualizations, and many other features that make Akooda stand out as an operational intelligence platform primarily focused on providing opportunities to make the most of business data.